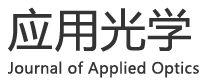
-
主管:中国兵器工业集团有限公司
主编:纪明
ISSN 1002-2082
CN 61-1171/O4
-
主办:中国兵器工业第二〇五研究所
中国兵工学会出版:《应用光学》编辑部
主管:中国兵器工业集团有限公司
主编:纪明
ISSN 1002-2082
CN 61-1171/O4
主办:中国兵器工业第二〇五研究所
中国兵工学会
出版:《应用光学》编辑部
机器视觉螺纹图像评价方法
Thread image evaluation method based on machine vision
{{custom_ref.label}} |
{{custom_citation.content}}
{{custom_citation.annotation}}
|
/
〈 |
|
〉 |