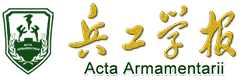
-
Sponsored by: China Association for Science and Technology (CAST)
Editor-In-Chief: Xu Yida
ISSN 1000-1093
-
Hosted By: China Ordnance Society
Published By: Acta Armamentarii
CN 11-2176/TJ
Loitering Munition Penetration Control Decision Based on Deep Reinforcement Learning
GAO Ang;DONG Zhiming;YE Hongbing;SONG Jinghua;GUO Qisheng
Sponsored by: China Association for Science and Technology (CAST)
Editor-In-Chief: Xu Yida
ISSN 1000-1093
Hosted By: China Ordnance Society
Published By: Acta Armamentarii
CN 11-2176/TJ
Loitering Munition Penetration Control Decision Based on Deep Reinforcement Learning
loiteringmunition / deepreinforcementlearning / Markovdecisionprocess / penetration / controldecision {{custom_keyword}} /
/
〈 |
|
〉 |