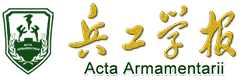
-
Sponsored by: China Association for Science and Technology (CAST)
Editor-In-Chief: Xu Yida
ISSN 1000-1093
-
Hosted By: China Ordnance Society
Published By: Acta Armamentarii
CN 11-2176/TJ
The Application of Ensemble Support Vector Machine to Fault Diagnosis of Diesel Engine Based onAdaBoost Algorithm
WANG Zi-ying;QIU Mian-hao;AN Gang;WANG Kai
Sponsored by: China Association for Science and Technology (CAST)
Editor-In-Chief: Xu Yida
ISSN 1000-1093
Hosted By: China Ordnance Society
Published By: Acta Armamentarii
CN 11-2176/TJ
The Application of Ensemble Support Vector Machine to Fault Diagnosis of Diesel Engine Based onAdaBoost Algorithm
{{custom_ref.label}} |
{{custom_citation.content}}
{{custom_citation.annotation}}
|
/
〈 |
|
〉 |