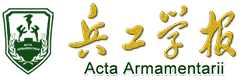
-
Sponsored by: China Association for Science and Technology (CAST)
Editor-In-Chief: Xu Yida
ISSN 1000-1093
-
Hosted By: China Ordnance Society
Published By: Acta Armamentarii
CN 11-2176/TJ
Improved GM-PHD Filter Based on Multi-target Uncertainty
WANG Kuiwu;ZHANG Qin;HU Xiaolong
Sponsored by: China Association for Science and Technology (CAST)
Editor-In-Chief: Xu Yida
ISSN 1000-1093
Hosted By: China Ordnance Society
Published By: Acta Armamentarii
CN 11-2176/TJ
Improved GM-PHD Filter Based on Multi-target Uncertainty
multi-targettracking / RFS / GM-PHDfilter / gaussianmixture / stateestimation {{custom_keyword}} /
/
〈 |
|
〉 |